Nonlinear Decision Rules Made Scalable by Nonparametric Liftings
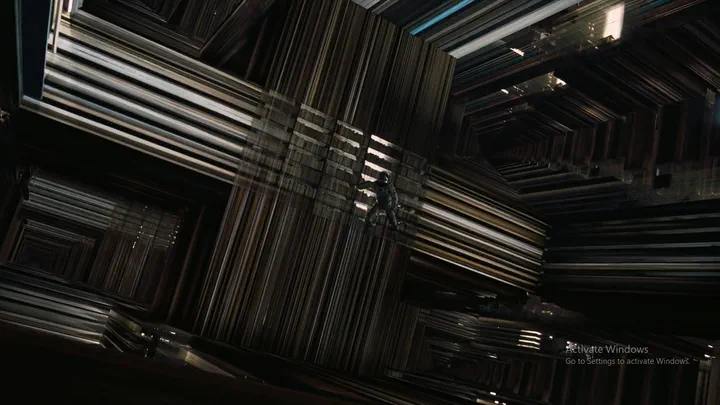
If you find yourself in a maze, escaping can be incredibly challenging unless you can view the maze and your position from a bird’s-eye perspective. Likewise, seeing things from a higher dimension opens up new perspectives and solution methods. This concept is also illustrated in the movie Interstellar, as you can see in the above, where Cooper goes into a black hole to send a message to his daughter in the past, leveraging the advantages of being in higher dimensions (I am not talking about the scientific feasibility of that scene!).
Analogously in mathematical optimization, the approach of treating sets as projections from higher to lower dimensions is known as lifting. This technique has been extensively studied in combinatorial and integer optimization, and more recently in robust optimization as well. In particular, existing lifting methods in dynamic robust optimization construct uncertainty sets in higher dimensions in such a way that the additional uncertain parameters can be interpreted as nonlinear (e.g., quadratic or piecewise-linear) functions of original uncertain parameters. These functions are chosen a priori and most existing lifting techniques are “parametric”; conceptually, they are similar to regression in statistics/machine learning.
In this article, we take an alternative approach that we label as “nonparametric” lifting, where we specify nonlinear functions to define lifting, with the goal of having theoretical performance guarantees while maintaining computational efficiency. This is done by utilizing information from optimization problems of interest, such as uncertainty sets, obejctive coefficients and constraints. These new methods demonstrate superior computational scalability, and these observations are magnified in multistage problems with extended time horizons, suggesting practical applicability of the proposed nonparametric liftings in large-scale dynamic robust optimization.